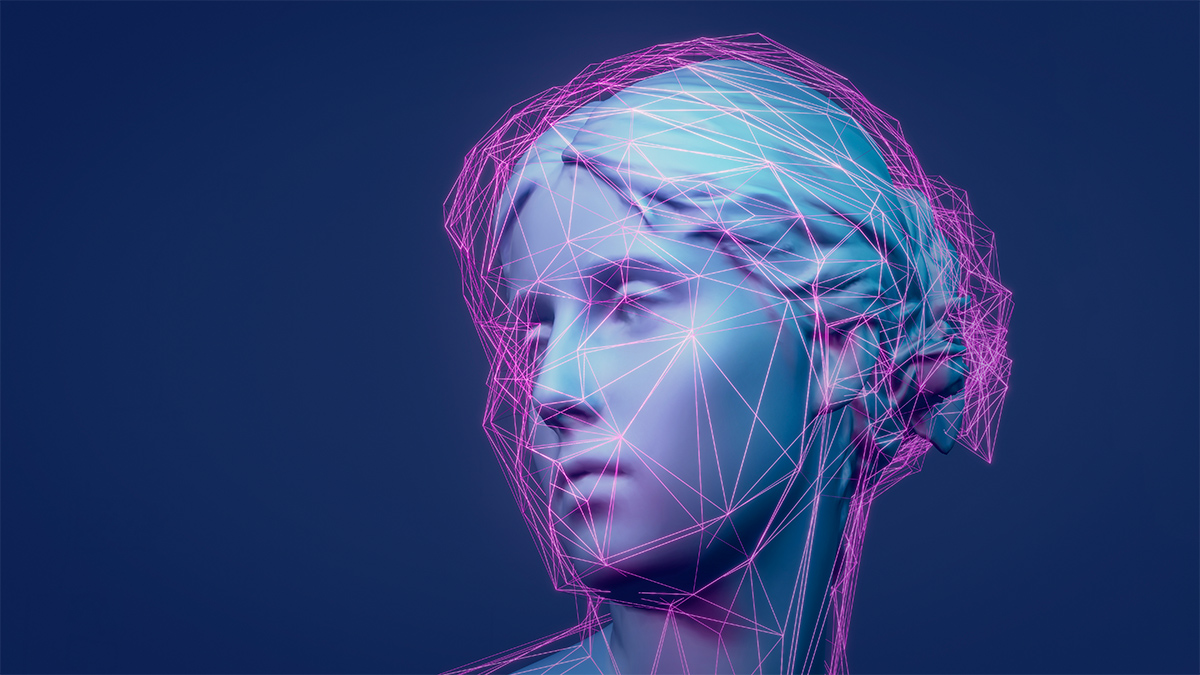
In an era defined by rapid technological advancements and the proliferation of data-driven decision-making, the ethical implications of machine learning have come under increasing scrutiny. From algorithmic bias and privacy concerns to transparency and accountability, the ethical considerations surrounding machine learning are complex and multifaceted. In this article, we delve into the key ethical challenges in machine learning and explore strategies for mitigating risks and upholding ethical standards in AI development and deployment.
Algorithmic Bias:
One of the most pressing ethical concerns in machine learning is algorithmic bias, where machine learning models inadvertently perpetuate or amplify existing biases present in the training data. These biases can manifest in various forms, including racial, gender, and socioeconomic biases, leading to unfair or discriminatory outcomes in decision-making processes. Addressing algorithmic bias requires careful consideration of data collection practices, model design, and evaluation metrics to ensure that machine learning systems are fair, equitable, and unbiased across diverse populations.
Privacy Preservation:
Privacy preservation is another critical ethical consideration in machine learning, particularly as the collection and analysis of personal data become increasingly pervasive. Machine learning algorithms trained on sensitive or personally identifiable information may inadvertently compromise individuals’ privacy rights, leading to concerns about data misuse, unauthorized access, and breaches of confidentiality. Implementing robust data anonymization techniques, encryption protocols, and access controls can help mitigate privacy risks and protect individuals’ sensitive information from unauthorized disclosure or exploitation.
Transparency and Interpretability:
The opacity of machine learning algorithms poses challenges to transparency and interpretability, making it difficult to understand how decisions are made and assess the rationale behind algorithmic outputs. Lack of transparency not only undermines trust in machine learning systems but also hinders accountability and oversight, particularly in high-stakes applications such as healthcare, finance, and criminal justice. Enhancing transparency and interpretability requires adopting explainable AI techniques, providing clear documentation of model assumptions and limitations, and facilitating stakeholder engagement and feedback to promote accountability and trustworthiness.
Fairness and Accountability:
Ensuring fairness and accountability in machine learning entails designing systems that prioritize fairness, equity, and social responsibility. This involves proactively identifying and mitigating biases, monitoring algorithmic performance for disparate impacts, and establishing mechanisms for recourse and redress in cases of algorithmic harm or discrimination. Additionally, fostering a culture of ethical awareness and responsibility within organizations, academia, and the broader AI community is essential for promoting ethical conduct and decision-making throughout the machine learning lifecycle.
Conclusion:
As machine learning continues to permeate every aspect of our lives, addressing the ethical considerations inherent in AI development and deployment is paramount. By prioritizing fairness, transparency, privacy, and accountability, we can harness the transformative potential of machine learning while safeguarding against unintended consequences and societal harms. Collaboration, interdisciplinary dialogue, and ethical reflection are essential for navigating the ethical landscape of machine learning and ensuring that AI technologies serve the collective good, uphold fundamental rights, and promote the well-being of individuals and communities worldwide.